Institute of Physical and Chemical Research(RIKEN) LLM development at the highest level in Japan. What are the challenges faced by a research team devoted to improving accuracy?
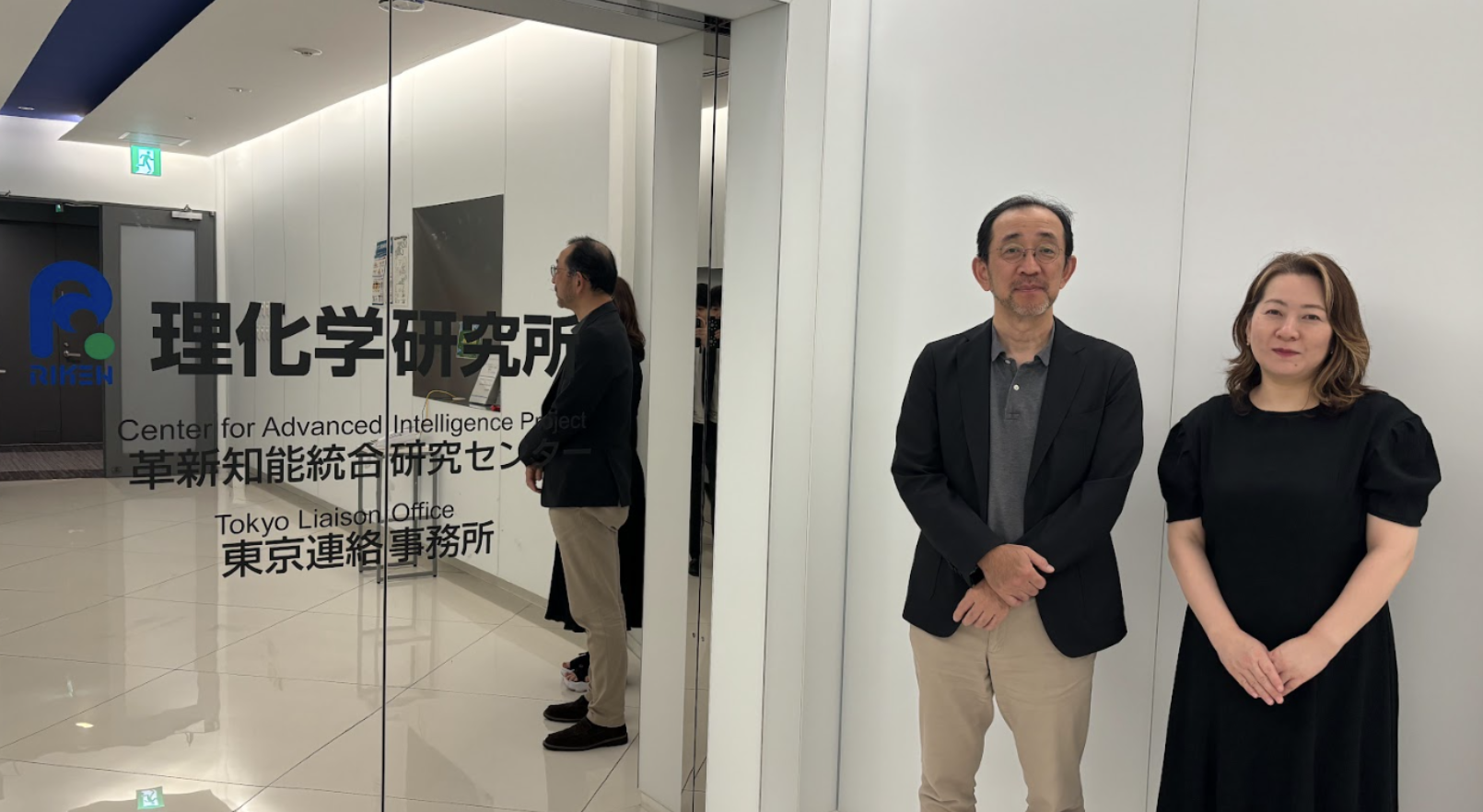
Thank you for taking the time to talk to us today. First of all, can you briefly introduce yourself?:
Mr. Sekine: I have been researching natural language for 35 years, and am currently involved in the development of a Japanese LLM at the RIKEN Center for Advanced Intelligence Project (RIKEN AIP). After graduating from the Tokyo Institute of Technology, I joined Matsushita Electronics (now Panasonic). After conducting various research there, I earned a doctorate from New York University and served as an associate professor.
During that time, I founded a company called Language Craft and served as the director of Rakuten's New York research lab.
Eight years ago, I worked as a team leader at RIKEN AIP to build structured knowledge, and in 2023 I started developing data for a Japanese LLM.
I am currently also a specially appointed professor at the Center for Research and Development of Large-Scale Language Models at the Institute of Informatics, and am conducting research and development on LLM.
Can you tell us about any current projects you’re working on?
Mr. Sekine: As you know, in November 2022 ChatGPT became available to the public. It had produced very accurate results on the project I’d been working on. When I saw the results, I thought, “I guess there's nothing left for me to do...'' and I thought about giving up on natural language research altogether. However, after that, I think it was in the summer of 2023, I was talking with a colleague and I started thinking, “I really need to make a Japanese LLM”.
That’s an interesting insight into the beginnings of LLM development.
Mr. Sekine: In a paper published by ChatGPT in March 2022, it said that instructional data was extremely important. In the research I was doing at the time, I had some very talented annotators around me, and I thought, "Maybe this team can create that kind of instructional data.” It was around August of last year that I started thinking about creating data, and trying to develop an original Japanese LLM and improve its accuracy. However, I didn't have any money at that time.
How did you raise the money?
Mr. Sekine: I tried making some and estimated that it would cost 20 million yen to make 10,000 articles similar to ChatGPT papers. So, I thought it would be a good idea to conduct joint research with about 10 companies at 2 million yen each. However, we actually received applications from 21 companies, and while conducting joint research with everyone we began creating data for 10,000 cases (Note: Joint research was eventually completed working with 18 companies). Initially, we had 15 annotators creating the instruction data, but we gradually realized that this was not enough, so we began placing orders with three annotation companies, one of which was APTO.
[Example of instruction data]
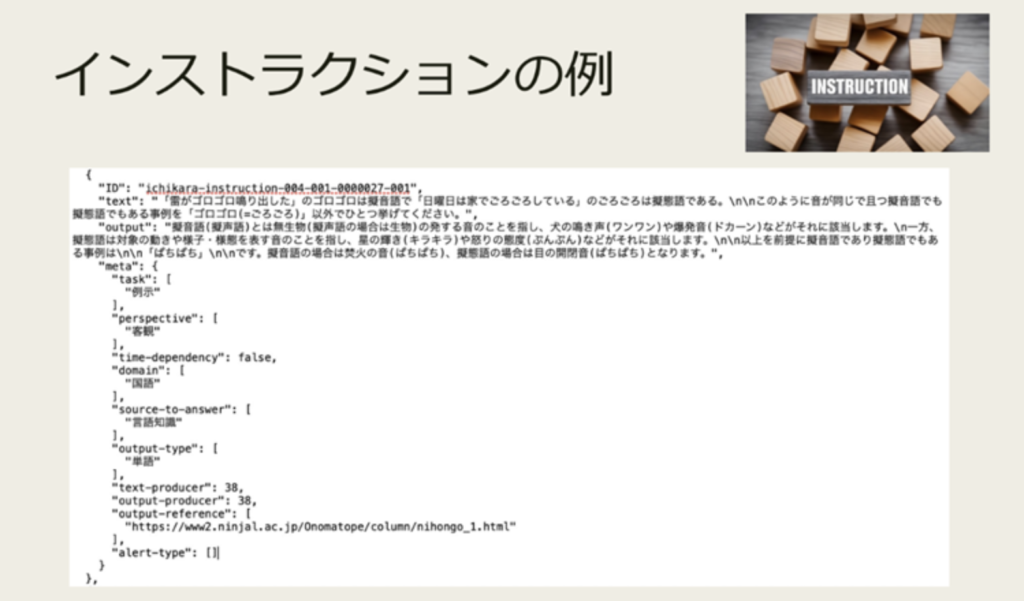
Reference: “The latest information and future of ichikara data” Satoshi Sekine (RIKEN AIP/NII-LLMC/Ichikara Co., Ltd.)
What challenges did you face creating Japanese LLM?
Mr. Sekine: First of all, we needed to produce high-quality data efficiently, but with a limited budget and resources. Creating instruction data is actually a very time-consuming task, even for those who are good at Japanese, and it is not something that just anyone can do. I realised that it would be difficult to obtain a high-quality Japanese LLM unless a trained annotator created a set of questions and answers, and another member carefully controlled the quality of the work.
[Creating a large-scale language model]
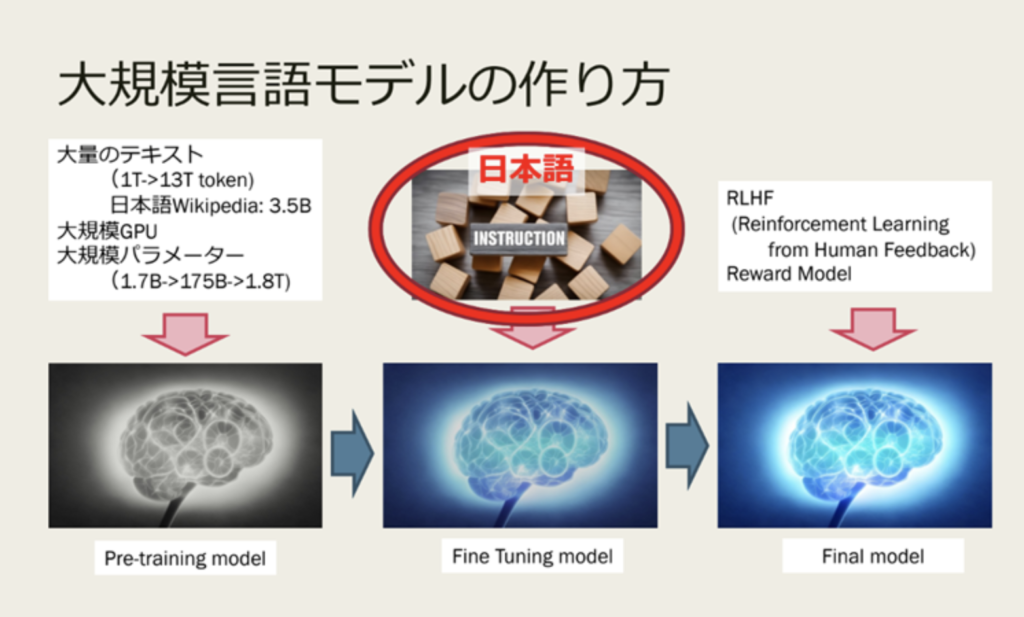
LLM model development can be divided into roughly three stages. The instruction data is used to fine-tune and improve accuracy.
Could you tell us a little more about the process?
Mr. Sekine: First, we create questions in a variety of categories. There are various types of requests, such as summaries (“Please summarize...”), examples, extractions (“Please extract...”), translations, etc. Answers can take many forms, such as sentences, proper nouns, programs, etc., and a certain amount of data for each category is required, which makes it very difficult.
Just hearing your explanation I can see how that would take a long time...
Mr. Sekine: This is something that APTO helped us with, and apart from a few examples of low detail, it was effective.
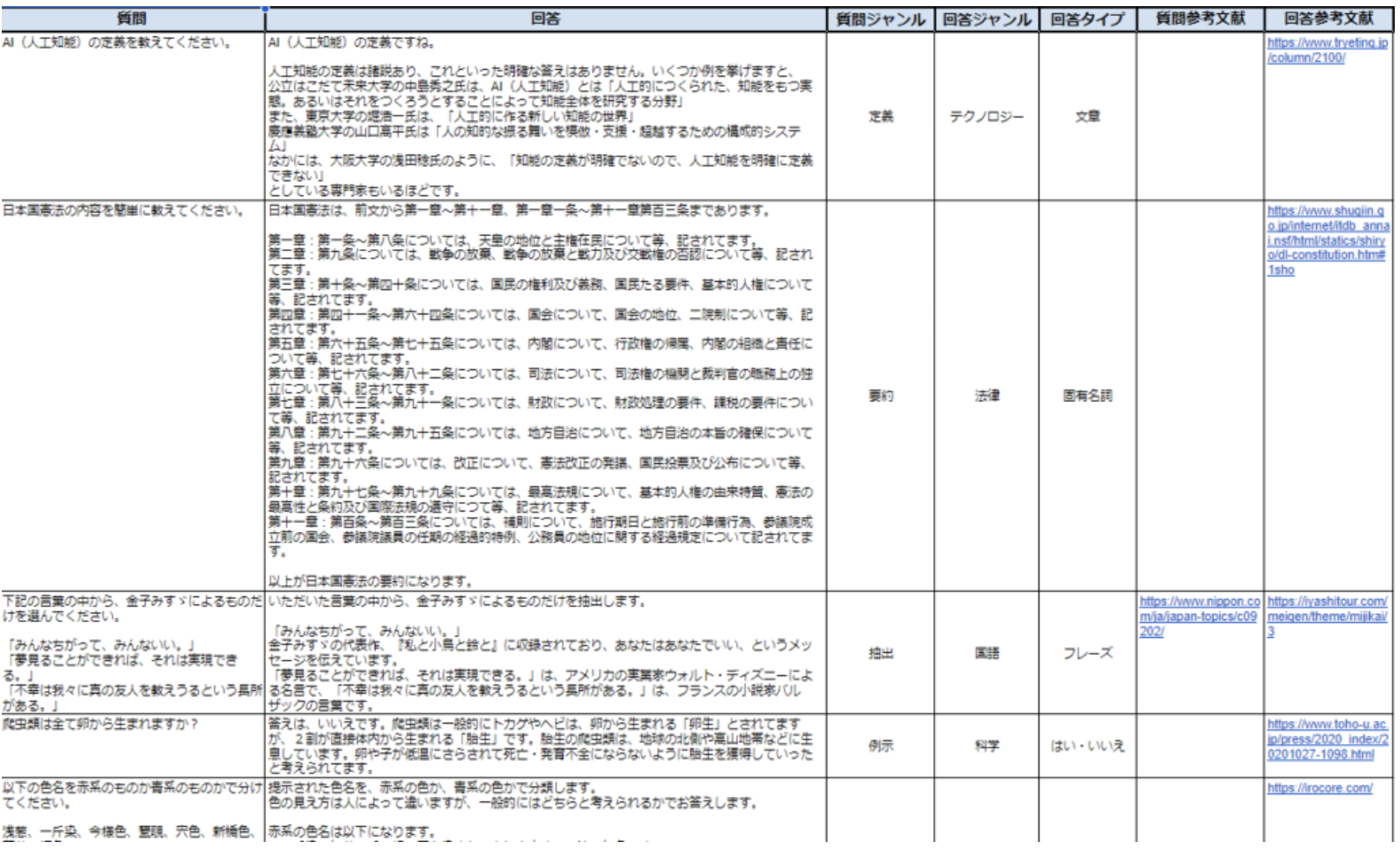
Some of the data created. Detailed rules are established for each Q&A.
It must have been difficult to gather the people needed?
Ms. Goto: It was! We explained to the outside companies we worked with, including APTO, the background of the project, the specific needs, the need for rapid production, and asked them to provide high-quality data in terms of accuracy. At the initial stage, we started without any specifications, but with the cooperation of everyone including APTO, we were able to efficiently create a large amount of data.
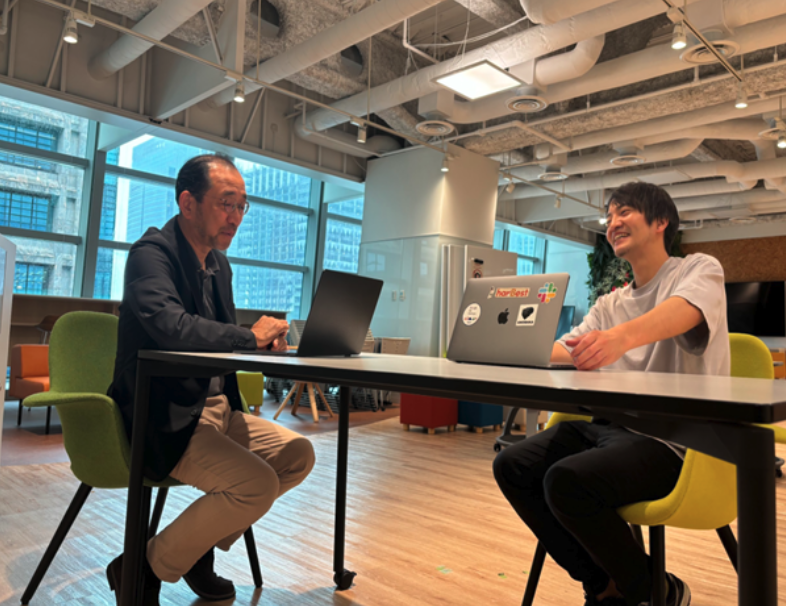
At the RIKEN AIP Open Space
How was the quality of the instruction data that was made?
Ms. Goto: Well, we started without any specifications so obviously the accuracy was not high initially. However, due to the great communication with APTO and because of your quality control and feedback systems, we were able to improve the accuracy very quickly and compiled the data in a short amount of time.
Mr. Sekine: Ultimately, with the help of a number of people, including APTO, we were able to create 10,000 pieces of high-quality data. This improved the accuracy of Japanese LLM and allowed us to build a more accurate model.
[YouTube link]
["Effects of Instructions in LLM Construction and Observations in Human and GPT-4 Evaluations" by RIKEN Professor Satoshi Sekine [W&B Meetup #12] Weights & Biases Japan]
We’re glad we were able to help! Could you tell us about your future goals?
Mr. Sekine: We would like to continue collaborating with APTO to create even higher quality data and improve the accuracy of Japanese LLM further. We would also like to work with APTO on some other projects and are aiming for further technological innovation. Building an LLM that specializes in Japanese is particularly important in gaining a deep understanding of Japanese culture, law, and social context. As we move forward with LLM development, I feel that we need to consider questions such as “Why do we need LLM?'', “What do we need it for?'' and “What kind of world are we aiming for?''. Since we still don't currently have enough data, we will build on a broader range of question types and collect natural question examples and multiturn and multimodal data.
We will continue to improve our harBest service, and we look forward to continuing to work with you.
関連事例
-
Search real estate all over the world at once using satellite data. What kind of future will “WHERE” make possible?
Penetrator
- IT/Internet
- real estate
- Annotation
- Data collection
- Data Management
- Experienced
-
“I started developing AI behind the scenes at a television station. Now I want to spread this throughout the company”
FUJIMIC, Inc.
- Annotation
- Data Management/Labeling
- IT/Internet
- Annotation
- Data collection
- Experienced
-
Achieve efficient form management by utilizing AI data. The secret to the success of ‘PATPOST’
ORIX Corporation
- Annotation
- Data Management/Labeling
- IT/Internet
- Annotation
- Data collection
- Experienced
-
Streamlining Development After Successful Outsourcing of High-Volume Annotation Work
The Ricoh Company, Ltd
- AI Development (Experienced)
- Annotation
- Data Management/Labeling
- IT/Internet
- Annotation
- Experienced
-
How Leading AI Vendors Handle Essential Training Data for Generative AI
LightBlue
- AI Development (Experienced)
- Annotation
- Data Management/Labeling
- IT/Internet
- Annotation
- Data collection
- Data Management
- Experienced
-
Micro Control Systems: AI “Visualizing” Factories to Enhance Manufacturing
Micro Control Systems
- AI Development (Experienced)
- Annotation
- Data Management/Labeling
- IT/Internet
- Annotation
- Data collection
- Experienced
-
harBest Boosts MiiTel’s AI Speech Recognition
RevComm Inc.
- AI Development (Experienced)
- Annotation
- Data Management/Labeling
- IT/Internet
- Annotation
- Data collection
- Experienced
-
Challenges of Developing “LHTM-2”, a Large-Scale Language Processing Model From Japan.
alt, Inc.
- AI Development (Experienced)
- Annotation
- Data Management/Labeling
- IT/Internet
- Annotation
- Data collection
- Experienced
-
AGRIST Interview: Developing Agriculture Technology to Support the Aging Farmers of Japan
AGRIST
- AI Development (Experienced)
- Annotation
- Data Management/Labeling
- Agriculture
- Annotation
- Data collection
- Data Management
- Experienced